Applied Linear Statistical Models Solutionsfreaksever
Applied Linear Statistical Models-Michael H. Kutner 2005 Applied Linear Statistical Models 5e is the long established leading authoritative text and reference on statistical modeling. For students in most any discipline where statistical analysis or interpretation is used, ALSM serves as the standard work. Applied Linear Statistical Models Fifth Edition. Download with Google Download with Facebook. Create a free account to download. Download Full PDF Package. Applied Linear Statistical Models Full Solutions Applied Linear Statistical Models Full Solutions - In this site is not the thesame as a solution reference book you buy in a collection buildup or download off the web. Applied Linear Statistical Models 5th edition is the long established leading authoritative text and reference on statistical modeling, analysis of variance, and the design of experiments. For students in most any discipline where statistical analysis or interpretation is used, ALSM serves as the standard work.

About
Applied Linear Statistical Models 4th Edition
This graduate level course offers an introduction into regression analysis. A researcher is often interested in using sample data to investigate relationships, with an ultimate goal of creating a model to predict a future value for some dependent variable. The process of finding this mathematical model that best fits the data involves regression analysis.
STAT 501 is an applied linear regression course that emphasizes data analysis and interpretation. Generally, statistical regression is collection of methods for determining and using models that explain how a response variable (dependent variable) relates to one or more explanatory variables (predictor variables).
Course Topics
This graduate level course covers the following topics:
- Understanding the context for simple linear regression.
- How to evaluate simple linear regression models
- How a simple linear regression model is used to estimate and predict likely values
- Understanding the assumptions that need to be met for a simple linear regression model to be valid
- How multiple predictors can be included into a regression model
- Understanding the assumptions that need to be met when multiple predictors are included in the regression model for the model to be valid
- How a multiple linear regression model is used to estimate and predict likely values
- Understanding how categorical predictors can be included into a regression model
- How to transform data in order to deal with problems identified in the regression model
- Strategies for building regression models
- Distinguishing between outliers and influential data points and how to deal with these
- Handling problems typically encountered in regression contexts
- Alternative methods for estimating a regression line besides using ordinary least squares
- Understanding regression models in time dependent contexts
- Understanding regression models in non-linear contexts
Course Author(s)
Software
This course uses Minitab statistical software. Students can use any software they wish for assignments, but most will find it easiest to use Minitab. Plus, examples for the course units will be demonstrated using Minitab. See the Statistical Software page for more information about obtaining a copy of Minitab.

This course uses Examity for proctored exams. For more information view O.3 What is a proctored exam? in the student orientation.
Applied Linear Statistical Models Dataset
Textbook
The textbook is required, and either of the two editions below is acceptable. Here are the two options for the required textbook for this course. Students may use either:
Applied Linear Statistical Models 5ed
The larger
- Applied Linear Statistical Models by Kutner, Nachtsheim, and Neter (5th edition)
OR the smaller
- Applied Linear Regression Models by the same authors, Kutner, Nachtsheim, and Neter (4th edition).

The first half of the larger Applied Linear Statistical Models contains sections on regression models, the second half on analysis of variance and experimental design. This first half of the 5th edition text is available published as Applied Linear Regression Models by Kutner, Nachtsheim, and Neter (4th edition).

Students may use either textbook listed as they are identical. Jimmy barnes double happiness rar extractor pdf.
The larger Applied Linear Statistical Models also includes 16 chapters on analysis of variance and experimental design not covered in this course, however, these topics are covered in STAT 502 where these chapters are required. Students may consider purchasing the larger text if they are taking both courses. Applied Linear Statistical Models is considered to be one of the 'bibles' of applied statistics so it probably will have value to you beyond this course.
Prerequisites
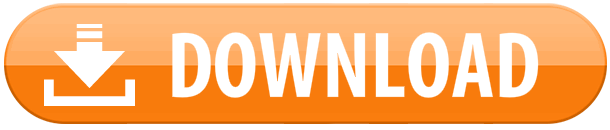